The technical work of the Berry Machine project was kicked off the previous year, with our interns running around the forests and calculating test areas. The weather was favorable and the atmosphere was positive, and by autumn we received a lot of material, about 10,000 images and hundreds of calculated test areas to be processed for Berry Machine.
In the summer of 2021, several cameras were used to photograph the berries; system camera and high- and low-end camera phones. Photos were taken from the top of the test plots and close-up photos for berry-free annotation. Using the acquired footage, the artificial intelligence was taught to identify different types of berries in the image: ripe, raw and in bloom. With the snow melting and spring already at the door, the year’s development work was already full. As the project progressed, its goals and needs in terms of machine learning began to become clearer, and the most important development targets were put under the magnifying glass.
Refinement of density calculation
Artificial intelligence determines the density of the berries using the berries visible in the pictures. Here, a linear regression curve is used, which is formed on the basis of the collected image and berry counting material. In practice, this means that the berries shown in the picture are multiplied by the coefficient of the curve obtained from the standard deviation, which varies according to the type of berries depicted.
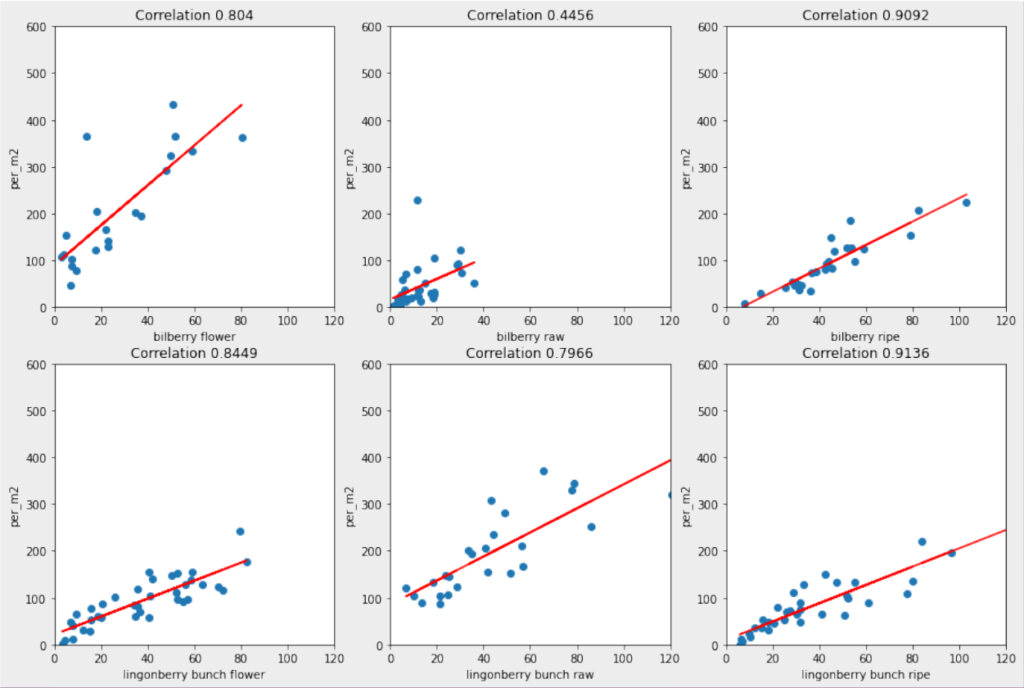
The berry machine proved to be an excellent recognizer for lingonberries and ripe blueberries, but blueberry blossoms and raw blueberries proved to be a challenge. According to our observations, artificial intelligence has difficulty distinguishing them from the background, especially when the images are wavering or overexposed, in which case it is difficult to look for them in the images even with the human eye. In addition, biases are caused by the place of growth; in the lushest places, the cover and shade of the leaves make it especially difficult to observe the raw blueberries. The above-mentioned factors make it difficult to create the most correlated coefficient for density calculation.
As you might expect, the help for studying the density calculation of less recognizable berry types is the larger and more enhanced data that we collected this summer. As a change from the previous summer, we also photograph smaller areas inside the test squares, which I hope will improve the algorithm in terms of density calculation. It is possibly easier to spot the berries in pictures taken closer. For filming, we prepared 50cm*50cm frames, which we use in connection with counting and filming test squares.
¼ frames are placed in the inner corner of the 100cm*100cm test plots and their berry amounts are counted separately and both are photographed from above and from each corner. The data from the small frames is combined with the data from the large frames, which can be used to compare how close the photographing affects the berry density.

The area of the images
Up until now, the area of the pictures has been determined based on the size of the berries. The program thus calculates the surface area of the image in relation to the pixel size of the berries in the image. With this method, the proportions of the pictures can be a bit off, as the size of the berries varies depending on where they are grown.
The determination of the surface area of the pictures taken with the berry machine was switched to using AR-based software, which is based on level recognition. In level detection, the artificial intelligence deduces the distance of the four points in the corners of the image and can use them to calculate the surface area of the image. A more accurate surface area calculation aims to promote the accuracy of the density calculation. The application works reasonably well in an office environment, but we hope to be able to test it in off-road conditions as the summer progresses.
Annotation
We added annotations with a set of 200 images, which mainly contains blueberry flowers and raw blueberries, to refine their identification. As an exception to last year, the labeling of lingonberries has been switched to marking only the bunches, as it was challenging to distinguish individual berries from the pictures.

The project people of Berry Machine wish you a good berry summer, which, based on the observations made in the forest, seemed to be really great!